The Importance of Quality Training Data for Self-Driving Cars
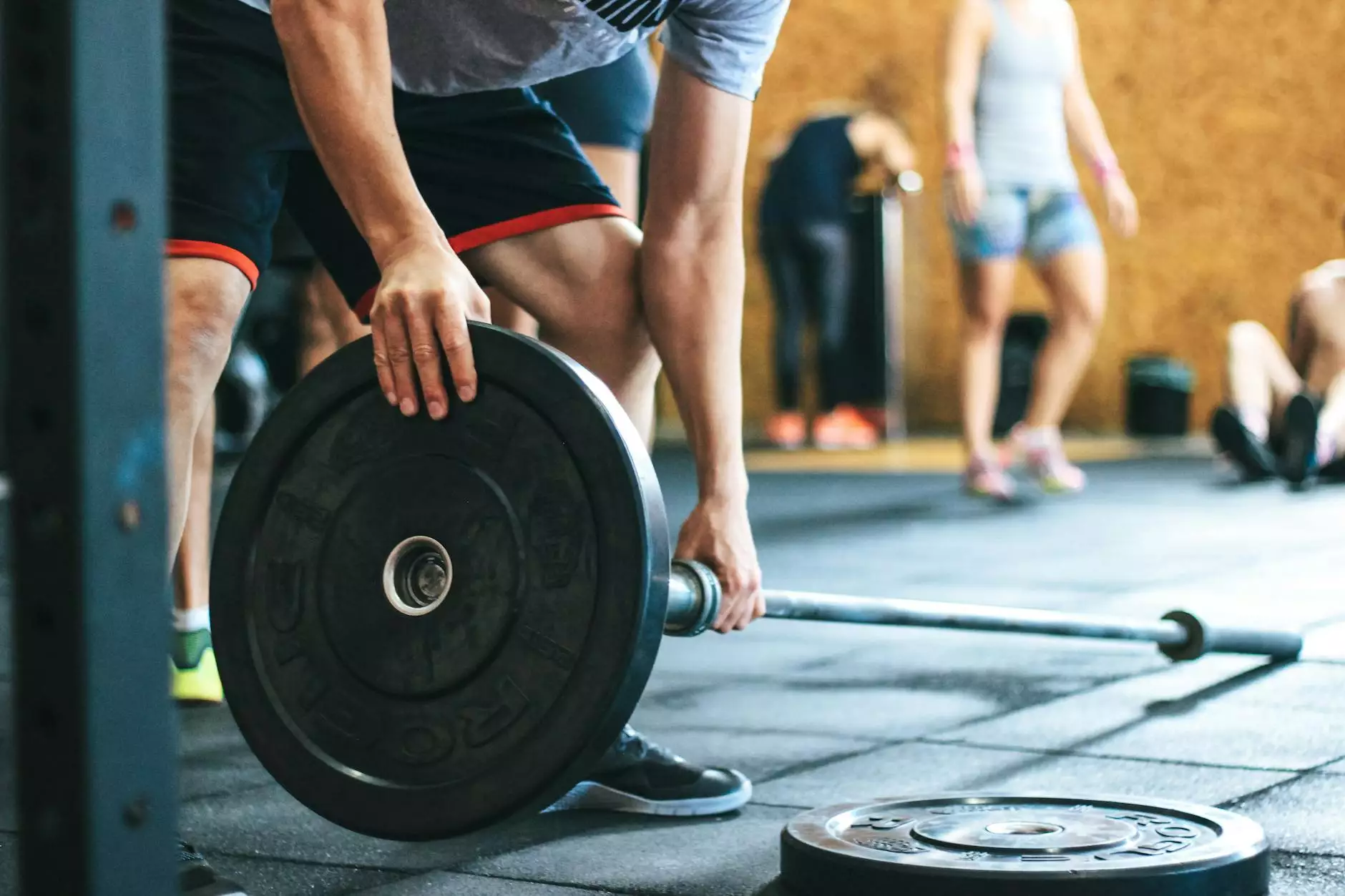
In the rapidly evolving world of technology, one of the most fascinating developments is the advent of self-driving cars. These vehicles promise to revolutionize transportation, enhance safety, and provide efficient mobility solutions. At the core of this technology lies a fundamental element: training data for self driving cars. In this article, we will explore the significance of this training data, how it is utilized, and the impact it has on the future of autonomous vehicles.
Understanding Self-Driving Cars
Self-driving cars, also referred to as autonomous vehicles, utilize a complex system of sensors, machine learning algorithms, and high-definition maps to navigate and control themselves without human intervention. The primary goal of these vehicles is to provide a safer and more efficient means of transportation.
Key Components of Self-Driving Technology
- Sensor Systems: These include cameras, LiDAR, radar, and ultrasonic sensors that gather data about the car's surroundings.
- Software Algorithms: Algorithms process information collected from sensors to make real-time decisions.
- Connectivity: Self-driving cars often communicate with each other and infrastructure to enhance safety and efficiency.
The Role of Training Data
Training data is the backbone of machine learning models used in self-driving technology. It is critical for teaching these models to recognize and react to real-world conditions effectively. In short, the better the training data, the more reliable and safe the self-driving technology will be.
What Constitutes Quality Training Data?
Quality training data encompasses a wide range of scenarios and experiences that a self-driving car might encounter. This includes:
- Diverse Environments: Data should come from various environments such as urban areas, rural roads, and highways to ensure the car can adapt to different driving conditions.
- Variety of Weather Conditions: Rain, snow, fog, and sunny weather can all affect driving; thus, data should include images and situations from all these conditions.
- Different Traffic Scenarios: This includes everything from heavy traffic jams to highway cruising, as well as interactions with pedestrians, cyclists, and other vehicles.
How is Training Data Collected?
Collecting quality training data is a meticulous process and typically involves several methods:
- On-Road Testing: Engineers use test vehicles outfitted with sensors to gather real-world data while driving in diverse conditions.
- Simulations: Advanced simulation technology allows researchers to create scenarios that may be too dangerous or impractical to replicate in real life.
- Crowdsourcing: Leveraging data from other vehicles on the road can help create a robust dataset for training algorithms.
The Importance of Data Labeling and Annotation
Once data is collected, it must be accurately labeled and annotated to be useful for training. This encompasses identifying and categorizing objects within the data, such as:
- Road Signs
- Pedestrians
- Other Vehicles
- Lane Markings
- Traffic Lights
This process is labor-intensive but necessary to train machine learning models effectively. High-quality annotations lead to better performance and safer autonomous systems.
Challenges in Obtaining Quality Training Data
While the importance of training data for self-driving cars cannot be overstated, there are various challenges associated with obtaining this data:
- Dataset Bias: If data is not diverse enough, it can lead to biased algorithms that perform poorly in underrepresented scenarios.
- Privacy Concerns: Collecting data, particularly in urban areas, must be balanced with the privacy rights of individuals.
- Cost: Gathering extensive amounts of high-quality data can be expensive and time-consuming.
Applications of Training Data in Self-Driving Technology
The applications of quality training data extend well beyond mere operation. They encompass several crucial aspects that contribute to the overall success of self-driving vehicles:
Safety Enhancements
Safety is paramount in the development of self-driving cars. By utilizing extensive training data, manufacturers can test and refine their algorithms to respond correctly to various situations, thus minimizing accidents. Continuous improvement in training data leads to improved decision-making processes within the autonomous systems, ultimately leading to safer roads for everyone.
Increased Efficiency
Training data also contributes to the efficiency of self-driving vehicles. By learning optimal routes, traffic patterns, and energy consumption behaviors through historical data, these cars can navigate roads in a time-efficient and eco-friendly manner.
Enhanced User Experience
Quality training data enables the development of systems that can recognize user preferences, adjust comfort settings, and deliver personalized services based on prior interactions. This enhances the overall passenger experience, making travel more enjoyable.
The Future of Self-Driving Cars and Training Data
The future of self-driving cars is promising, and as the technology evolves, so too will the methods of collecting and utilizing training data. Future advancements may include:
- AI-Driven Data Collection: AI could automate the gathering and labeling of training data, improving efficiency and accuracy.
- Improved Simulations: As technology advances, so will the realism of simulated environments to test self-driving algorithms.
- Better Collaboration: Partnerships between tech companies, automotive manufacturers, and government entities can lead to better data-sharing practices.
Conclusion
In summary, the importance of training data for self driving cars cannot be understated. It is the foundation upon which the safety, efficiency, and effectiveness of self-driving technology are built. By understanding the components, challenges, and future potential of this data, we can appreciate how it shapes the autonomous vehicles that are likely to be a regular aspect of our lives in the years to come.
As we continue to explore the advancements in autonomous driving technology, it is crucial to focus on enhancing the quality and diversity of training data. This will ensure the safe integration of self-driving cars into our transportation systems, paving the way for a more efficient, accessible, and safer future.